I’m going to do a series of posts here on rethinking LLMs around some key concepts and terms in the discourse: hallucination, context, citation, etc. I’ve spent some marvelous time this summer exploring British Columbia, Florida’s panhandle and otherwise, and I’ve tempered some of my thinking around artificial intelligence in education, so I feel a little less of the polemic urge. But I’m going to introduce this series below by fleshing out this tweet I dropped for a friend earlier today:
Dichotomous discourse
There’s a lot of.. discord in the discourse. That’s cool, and that’s mostly what I study: how people talk, argue, agree, disagree, utter, etc. This positions me well to report to you a few things about AI discourse that you might already know, but are worth further examining and clarifying.
There’s this absolutely fantastic paper I found from 2023 that gives a great framework through which to examine the AI/LLM discourse; it’s called Discourses of artificial intelligence and there’s an open PDF, so check it out. A few critical pieces illuminated there in my view are dichotomies about human vs. machine and utopia vs. dystopia:
"The first dichotomy — utopia-just-around-the-corner versus dystopia-is-now — aligns with the doomster/booster dualism noted by Selwyn (2014). The second and, possibly an even older, duality is of human versus machine, invoking the mythic language historically associated with new technologies over time (Mosco 2005)."
In my wonderful, sublime, glacial reflections, I took note of my own tendency to monologize and join the utopia-just-around-the-corner crowd. But that’s not what I’m going to do anymore. So here’s my tip for you: If you’re encountering the commentariat and you want to keep a skeptical lens, consider if it’s selling you a solution for everything, or dismaying that the entire edifice of ed is collapsing.
Neither of those are true. That means Sal Kahn isn’t going to solve all learning through his digital academies, but neither are kids using LLMs for assignments going to rot their brain through melodramatic cognitive atrophy. Anybody telling you either of these is full of crap—and that’s also discussed in the review above as limited empirical research on AI’s actual impact.
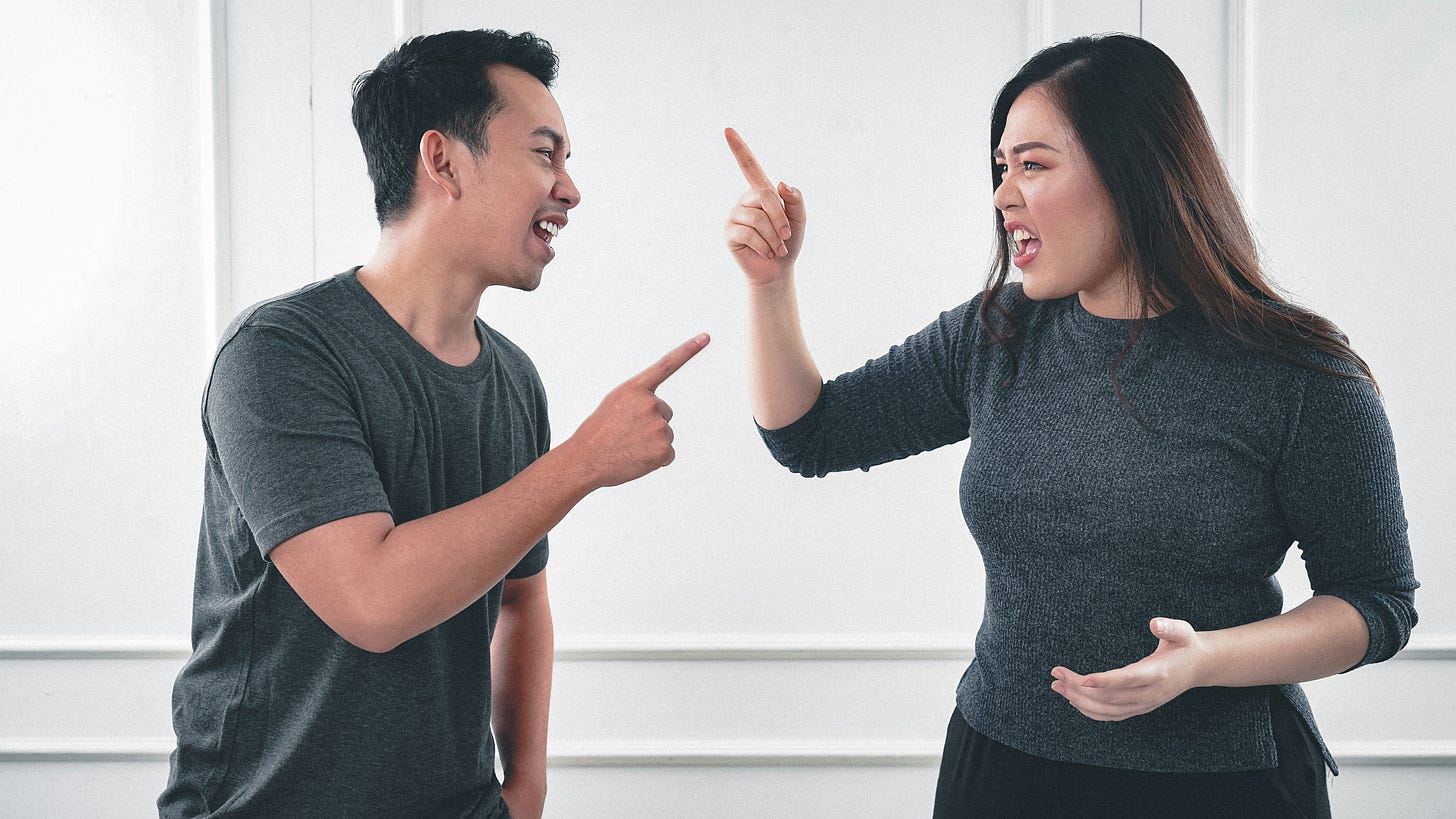
LLMs aren’t AI writ large
Another thing people do in the AI discourse all day long is conflate LLMs with AI broadly. I get that this happens from the hypester/utopia folk and the doomster/dystopia folk—and OpenAI doesn’t help out here with its pursuit of general artificial intelligence (easily confused with generative artificial intelligence, a different thing).
What I mean here is that there’s an active conflation of “language model” capacity with chatter about all manner of AIs—recommendation engines, planning algorithms, and decision-making systems are all types of artificial intelligence that don't rely on large language models. Yet critiques of emergent LLM capacity typically play on these tropes, one’s that seem to emphasize the lack of a human in the loop (automation), or the diminution of thinking (outsourcing).
A cool way to catch people out with this one is a neat little dictum that came to me: language models aren’t thinking models. You’ve probably seen a lot of “AI in ed critics” joshing about their newest puzzle prompt that stumped an LLM. Let’s remember that LLM stands for large language model, not large thinking model. Expect the models to exercise their linguistic expertise, not their thinking expertise. This is like criticizing a word processor for being bad at taking pictures.
Language models aren’t thinking models!
Text processing
By the way—that’s not a bad conception for LLMs: text processors. I’ve seen it argued before: we’re used to word processing tech (including AI with spell check and autocorrect), but for some reason with text processing tech we’re expecting it to perform fantastically intelligent feats of conceptual thinking. I understand that bragging and boasting and speculating about emergent “intelligent” or “rational” capacities with LLMs is part of the business model of big corps out there, but the critics don’t have to uptake this and mislead people.
So my tip to you? Name whether you’re talking about AI writ large or LLMs. Remember that LLMs are language models, not thinking models. So employ them to do language transformations or tasks, not thinking tasks. When someone uses an LLM to summarize a text, they’re not outsourcing their thinking, they’re giving a tool a language task. They’re thinking the whole time they bring the text to the model, prompt the model about it, and interpret what the model generates as a result. Conceiving of this relationship between humans and tech tools as adversarial is a false dichotomy.
In fact, people just love to cite this other paper Language is primarily a tool for communication rather than thought to make fun of LLMs that can’t think, but folks are missing the point there as well. This paper makes a convincing case that we can think without language. So why do people interrogate a language model for evidence of its thinking? Why do some insist that writing a text is the best way to think? Or that a learner must produce a written text to express their thinking? Once you see this incoherence in the critique, you can’t unsee it. It’s fun, in a way— if also totally frustrating all the time.
So what?
So stick with me over the next few months as I try to demystify the discourse on LLMs. Keep in mind these distorting dichotomies as you go forth into a new academic year: LLMs won’t usher in a total learning utopia, nor will they destroy learning institutions through cognitive automation. LLMs don’t operate without a human in the loop, so there is no sensibility to human vs. machine—and to be frank I wish people had more faith in the dignity of their fellow humans there. Now, next up? Hallucination.